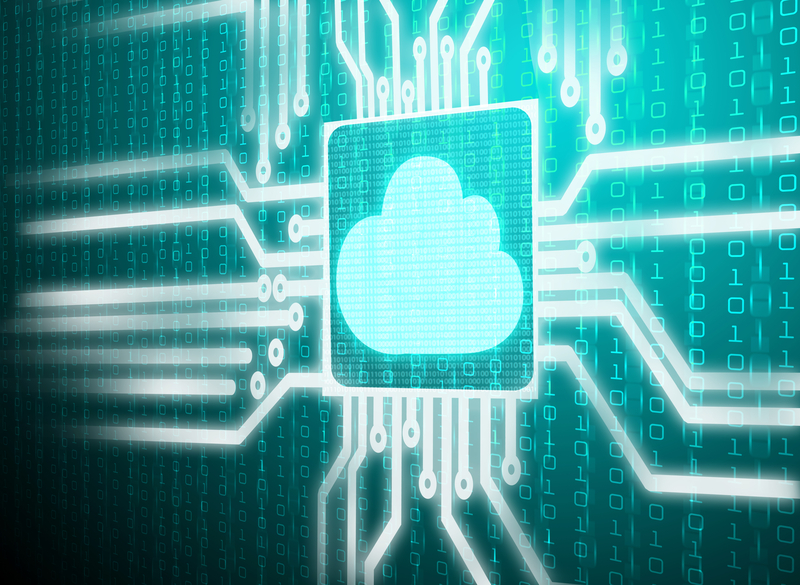
Globally, railway infrastructure operators share the same ambitions. Broadly, these are to derive more capacity and efficiencies from existing infrastructure through the application of a greater range of data-derived applications. At the same time, safety and security must remain un-compromised; better yet, they should if possible be significantly improved.
Digitalisation is the means of delivery. Unfortunately, the railway sector faces the same problem which bedevils other modes of transport: the ambitions might be identical but developments have taken place in geographically isolated pockets. In many or even most instances, little or no regard has been paid to the realisation of physical links or protocols which enable interaction between the different deployed solutions.
Earlier-generation solutions to such issues turned to the internet. For example, starting in the early 2000s, with the aim to achieve strategic management of the whole of the Trans-European road network, the Datex2 standard for cross-border information exchange was developed. National and regional roads operators and service providers in the European Union have since coalesced around its use.
Datex2 presents information in XML format. Infrastructure operators post to a common web location and those parties with appropriate permissions can download that information for interpretation and use in their own traffic management systems and services.
The standard was a pragmatic solution to what could have been a horrendously complex and costly engineering exercise to hard-wire connections between traffic management centres in numerous different countries.
Nevertheless, Datex2 still resulted in a bespoke solution for a relatively limited number of users in a single sector: roads.
Enter the cloud
Coming forward a generation, the cloud has emerged as a reliable means of unifying and managing data from large numbers of sources that can sometimes be significantly different.
A key differentiator for the cloud is that it has universal utility, in the sense that a single technological solution can be applied to different end uses.
Not only does this make deployments simpler, quicker and less costly, it also means that as different sectors come together they can be united more readily via the same platforms. This is a significant enabler of, for instance, Mobility as a Service, which aims to provide travellers with information on journey options across multiple modes but which, to be successful, relies on the integration of data from many different stakeholders.
Just how readily cloud-based solutions can adapt to address different industries’ needs is illustrated by the launch in mid-2024 of Huawei’s Pangu Railway Model. Used in concert with the Huawei Trouble of moving Freight Car Detection System (TFDS) to address the challenge of inspecting wagons more efficiently and with greater levels of consistency, this represents another significant step forward in the use of Artificial Intelligence (AI) in the railway sector.
Automating inspections
The Pangu Railway Model can accurately identify 67 types of freight cars and over 430 types of faults in corresponding specifications. It can quickly analyse millions of images captured by the TFDS and filter out more than 95% of the images that are fault-free. For the rest of the images, the AI system can mark the faults and train inspectors can focus on these and review the marked faults.
In the railway sector, fault identification is an area that has typically relied upon manual image inspection. Automating the process through the application of machine learning/AI vastly reduces the number of images that need human interventions.
The reduction in effort is significant. Taking as an example a typical train of 50 wagons and around 80 image captures per wagon, 4,000 images per train are generated. In a railway depot which inspects 10 trains per day, that results in 40,000 images.
This AI-based solution has revolutionised the fault identification process, guaranteeing safer railway operations and enhancing reliability; the result is a fault detection omission rate of almost zero, and a correct rate of fault identification as high as 99.3%.
The Pangu Railway Model uses continuous learning to improve performance. If abnormalities are detected, they can be manually labelled, and added to the model to improve detection performance.
The Model also supports concurrent detection on distributed servers, significantly reducing the time required for fault detection. Using a two-node solution, the detection process for a train is completed within eight minutes by one person. This compares to a time-constrained 15 minutes for fully manual inspections by a team of four. The AI solution could greatly increase the efficiency of fault detection.
The faster detection process therefore ensures timely identification of faults, mitigating risks and enhancing overall railway safety.
Railway Cloud Solution
At the ground level, the increasing use of the 4/5G-based Future Railway Mobile Communication System is already bringing us many of the changes that are being demanded by both train operators and users. These include moving block-based train operations, real-time train status monitoring, intelligent operations and maintenance, and railway IoT, as well as value-added services like multimedia dispatch communications, intelligent infrastructure management, trackside IoT, predictive maintenance, and passenger information transmission.
All of this leads to railway networks that function far better in all respects. However, it also leads to far greater quantities of data that need to be effectively managed in real and near-real time; if relatively timely and technologically less complex deployments of new operating methodologies are to be achieved across larger geographic areas, the cloud is the only realistic solution.
The Huawei Railway Cloud Solution provides all of the features described above. It has been developed specifically to address the industry-wide challenge of inconsistent standards across platforms and optimisation of resource utilisation while enhancing efficiency and assuring reliability.
Architecture and features
The Huawei Railway Cloud Solution is a distributed design that features three-level collaboration at the railway group, railway regions and edge depot levels.
Building the architecture in this manner results in a cloud solution that is secure and reliable. It also becomes much more resilient: the group centre adopts geo-redundant disaster recovery, meaning that mission-critical components can be distributed across multiple servers. The railway administration centres can construct their own back-up centres or re-use the resources provided by the group centre. Meanwhile, edge computing nodes at individual depots are interconnected through the data communication network.
The Huawei Railway Cloud Solution integrates hardware and software to provide a full-stack solution — computing, storage, networks, operating systems, databases and platforms. Its multi-scale (local/intra-city/remote/geo-redundant) and all-scenario disaster-recovery characteristics meet the different reliability requirements of all levels of railway operation.
The solution’s developers have ensured that a mature toolchain and ecosystem for adaptation and migration, combined with excellent end-to-end services and best practices, enables quick adaptation of railway applications and smooth migration to the cloud.
PRASA realises benefits
Huawei’s cloud stack is already providing the Passenger Rail Agency of South Africa (PRASA) with a unified application deployment platform.
The PRASA deployment utilises ManageOne, which provides centralised control, governance and Operations and Maintenance (O&M) capabilities; because one tool is used to cover all O&M functions, efficiency is improved and there are reduced labour costs.
Use of the cloud stack has put an end to siloed activities. Looking forward, it will enable more rapid application deployments and so greatly improve production efficiencies — the Rail Cloud Solution’s quickly configurable resource allocation reduces the time taken to launch apps from months to just a few days, effectively making it an on-demand service.
Summarised benefits
By comparison with historical methods of working, the Huawei Railway Cloud Solution improves resource utilisation by at least 100%. Its geo-redundant, intra-city active-active and application active-active disaster recovery capabilities ensures the reliability of key services; active-active applications based on cloud native services and databases achieve a recovery time objective of less than 60 seconds and a recovery point objective of zero. Software and hardware synergy improves big data performance by 30%, and the capabilities of the Pangu Railway Model significantly accelerate the development of new AI-based applications.
Content produced in association with Huawei