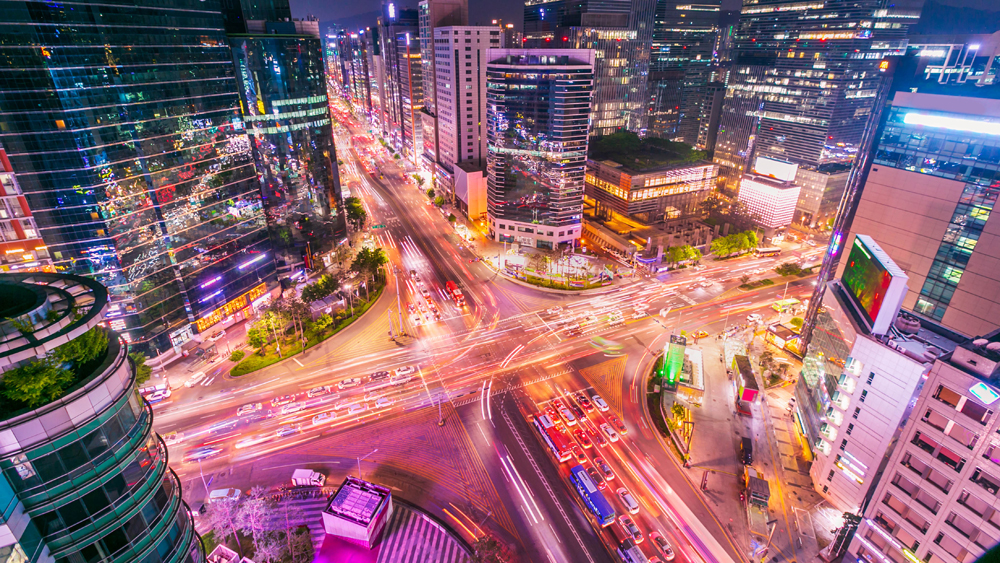
Artificial intelligence (AI) has the potential to improve transport and serve as a major component of future smart cities, but Here Technologies says it can also help solve a more thorny issue: privacy.
Speaking at a panel discussion at CES 2020 in Las Vegas, Giovanni Lanfranchi, Here’s chief technology officer, envisaged a future in which location data in a city is “private by design”.
“Secondly, I believe in personalisation, so what I like may be different and my city location behaviour can be personal,” he said. “Thirdly, a community could have transparency on where things are and there may be a lot of use cases in transportation and automotive.”
However, Lanfranchi recognises location from a privacy standpoint is ‘very tricky’ because it has a spatial and temporal sequence – it can reveal the place and time that the information is taken.
“That’s why our blockchain-based consent management and Anonymisation as a Service are key elements to give the data subject the ability to decide where to share and for what purpose,” he added.

However, panel moderator Ina Fried, chief technology correspondent at Axios, highlighted that AI is not allowing computers to create and think but to do things that humans process at a large scale.
Also, they are only as good as the data they receive which can lead to bias; an issue which Lanfranchi said Here is responding to by working with “the power of simulation to avoid or minimise”.
Simulation is also used alongside the company’s autonomous vehicles (AVs) to gather data, and Here researcher Dr. Michael Kopp believes this mix is going to continue.
“I think you’ll see it a lot, mostly because one of the artefacts that this wave of AI has washed ashore is the success of reinforcement planning,” he told the audience at CES 2020.
“Anything with a control theory flavour, you could potentially tackle by having a simulation or having AI self-train in simulation,” he said. “That simulation has to be very good so in most cases, you would want to machine learn it, if you like.”
Open simulation
Lanfranchi emphasised another important area for AI relates to ‘open simulation’, a method which he relates specifically to a competition carried out to predict traffic in Berlin (Germany), Moscow (Russia) and Istanbul (Turkey).
The Traffic4cast competition merged ‘movie-prediction’ machine learning (ML) with traffic research to provide competitors with an opportunity to understand complex traffic systems and make predictions about how they would flow in the future.
Kopp – also director of the Institute of Advanced Research in Artificial Intelligence – explained that Here took probe data from the cities and mapped them into 100 x 100 grid squares and aggregated the speed, volume and average heading for every five minutes.
“Essentially, we get a very jittery movie, and the question was: ‘If we give you 285 days’ worth of movies in 2018, can you predict on the remaining days? If we give you an hour’s worth of movie, can you predict the next three frames?’” Kopp continued. “The neural networks we used manage to capture the component that was temporal and somehow they were very good; if you took them sequentially they could fill in the gaps much better than we thought.”

“We got some insights on how to have a better fleet management for large operators to optimise fleet and reduce CO2 emissions,” Lanfranchi added.
During the same session, Enabling an efficient mobile future with location-based AI, Lanfranchi underscored the importance of users being able to understand how their data is being used while retaining the right to be forgotten.
“As an OEM [original equipment manufacturer], what I want is not to have all anonymised or all non-anonymised,” he elaborated. “I want to have the ability to manage the risk between how much data I am providing may be subject to some conflation and misuse versus ‘well if I randomise too much at the end of the day it’s just garbage’ - that’s the trade-off.”
Four data points
Kopp refers to an external study in which de-anonymised data shows that data on four places that a vehicle has travelled is enough to reveal the driver in 80% of cases – prompting the question of how to get useful data that is not personal.
“At the moment I believe no one has an answer, and I think what makes it possible to de-identify is that big data tooling is ubiquitous” Kopp admitted. “You have with AI and other ML techniques a powerful tool to de-identify people but on the other hand with blockchain you have other technology that can help you prevent it. You can also use AI again to make it anonymous, so you have essentially an arms race going on.”
Lanfranchi thinks it’s important to look at each use case because the trade-off between anonymisation and non-anonymisation needs to be “on the riskier area” for a location-based advertisement to understand that someone has done shopping at some point.
“But if I am on HD mapping and I just need to understand that there is a barrier, or a new construction zone or roadwork, honestly I don’t need to know when you started or when you arrive,” he explained. “I just need to statistically to have enough data to construct the new feature or barrier that has just been put at that particular point.”
When asked to specify the key obstacles on this journey, Kopp claimed there are very few examples taking place at the moment where ML - or the promise that these models give - is being put into production.
“I think successfully doing that at scale is something people are struggling with; anyone who builds an autonomous car will have that problem and people like us who look at fleet management,” he pondered.

Looking ahead to CES 2021, Kopp predicted the open nature in which AI needs to be developed will be a hot topic of conversation.
“AI these days is black box: we know how it works but we don’t know why it works. Trial and error on an industrial scale is the production method and there’s nothing around it,” he concluded.
Advances in AI and ML certainly appear to be ticking a lot of boxes for their relevance to the ITS space while also providing much-needed assurance that data remains private, but the need for the level of collaboration Kopp is referring to could well be the catalyst for real change.
AI, ML and privacy
Speaking to Lanfranchi at Here’s booth, the chief technology officer delved further into the usefulness of the technology by claiming it is “completely impossible” to create an HD map without AI or ML.
“So, one dimension is to really leverage machine learning to automate the creation of the map along with a far better accuracy in the centimetre range and into a fresh cycle,” he told ITS International.
He explained that Here has also been using AI and ML to provide a “semantic context” around location. “One example would be routing, we as humans we do not navigate following a set of very specific and rigid turns, but we say ‘Oh okay I recognise this landmark and maybe I need to turn’ and we want to replicate into our services and this can be done through AL and ML,” he added.
Additionally, the company has also developed a consent management component to its Here Marketplace as part of a “strong privacy focus”.
Here Marketplace is a platform that offers a neutral environment to facilitate data exchange between consumers, providers and third parties. Consumers can use the hub to exchange information across industries as well as create and license location-centric data and services. It also allows companies to share sensor data with other parties to create location-based applications and solutions for individuals and enterprises.

Lanfranchi revealed that the consent management component of the marketplace can allow an insurance company to leverage the data to build an insurance premium application.
“The problem is that we need to have the consent management part so that whoever is using the app can provide explicit consent that their data will be used for that specific purpose,” he continued. “We have built this consent management component on top of a blockchain distributed ledger because that’s the only way we can ensure trust across parties who do not necessarily trust each other.”
This component also allows an individual to change their mind at any time and no longer allow their data to be used for a specific purpose.
Within consent management, Here has developed a neutral server component to assist European OEMs who are required by the European Commission to publish all safety-related data.
“The neutral server allows everyone to access a neutral point and leverage all this safety-related data in a non-discriminatory condition and the OEM does not even know who will be the player who will use this data,” he beamed. “This is achieved through our consent management neutral server component that we built on top of the marketplace.”
Delivery influx
AI and ML are not the only things on Here’s mind. Jørgen Behrens, senior vice president, head of apps & services told ITS International how Here can also help cities cope with the increasing amount of delivery vans clogging up their road network.
“In a city like Amsterdam, you see delivery vans bringing packages to residential addresses in unbelievable numbers and driving is becoming replaced with shared mobility,” he begins.
To combat this issue, Behrens revealed the company is releasing Here Last Mile to help last-mile delivery fleets optimise their work planning.

“So if a company with 100 vehicles needs to deliver 10,000 packages, how do you optimally distribute the packages across the vehicles so you drive the least amount of miles using as few drivers and vans as possible?” he continued. “We’ve kind of packaged that up in an end-to-end application with a dispatcher interface and a driver application that solves that whole problem.”
He claimed that a fleet’s challenges on how to plan routes and the allocation of packages most efficiently can be done through Here’s Matrix Routing algorithm.
“If you run the same number of jobs with the same parameters of how many vehicles you have, and their size and range and all of this through our algorithm, it produces more efficient routes than other solutions do.”
In the development of the solution, Here also considered feedback from delivery drivers who unlike consumers “spend almost their entire time travelling in smaller roads where conditions are just different and the level of information available to you is also very different”.
Cities are also presenting a whole other range of issues for delivery drivers such as closing roads, installing barriers that only local residents can travel over or making streets ‘bicycle-only’.
Commenting upon what cities can do to help, Behrens believes in the importance of publishing data on changes to the road network more proactively.
“Many cities, especially in Europe, are old and investments are constantly being made in upgrading roads and replacing gas lines and pipes,” he elaborated. “The better they get at publishing that information and doing it in a consistent manner, that will help us make our traffic data better.”
Looking toward the next steps in last-mile delivery, Behrens warned of a need to take the “systemic nature” of the situation by looking into whether there is a way for packages to be redistributed during the day between vehicles.

“The ability for multiple logistics providers to collaborate is something that a city could start a conversation about, especially a major city that has a lot of logistics going on,” he pondered. “So is there a way to optimise the system even further by vehicles meeting each other and exchange packages to achieve a more efficient flow of logistics; that would be something quite interesting to work with.”
The influx of delivery drivers swarming in and out of cities does not appear to be wavering any time soon, underscoring the need for a solution. Here Last Mile may prove to be an efficient tool in managing a fleet while also pointing to a brighter future in which providers can collaborate on a shared approach.
Traffic4cast competition
Here Technologies says results from the Traffic4cast competition show how AI can effectively uncover insights to solve traffic gridlock through trial and error of its industrial geospatial data. Effectively identifying and analysing traffic patterns is expected to lead to more accurate predictions of how traffic would move on given roads at given times of the day.
The company claims computer systems modelled on the human brain, called neural networks, can help because they learn to carry out tasks by considering examples, such as datasets, usually without being programmed with task-specific rules.
Traffic4cast results revealed that neural networks were the most effective method for predicting traffic and came closest to simulating the exact traffic flow. Winners from South Korea, Oxford/Zurich and Toronto were among more than 40 teams from around the world who submitted more than 4,000 entries.
For the competition, movie clips were created using data based on more than 100 billion probe points from positions reported by a fleet of vehicles which captured morning, evening and rush hour traffic. Each movie frame summarised GPS trajectories mapped to spatio-temporal cells. The movies showed multiple colour channels characterising traffic volume, speed and direction.
The participants forecast the traffic by completing the next part of the movie clip for all three cities. They were given 285 full training days (full movie for the entire day) and 72 testing days (five blocks of 12 consecutive images with at least 30 frames between each block) while the rest were marked- out validation sets. Each contestant then had to produce three consecutive images following each given block of 12 images in each movie file for each day in the test set for each city.
Keeping the vehicle in lane
Here is also offering a digital representation of the global road network which helps a vehicle to position itself in a lane while providing drivers with lane-level visual guidance.
The company says Here Lanes feeds advanced driver assistance systems and mobile applications with lane topology, geometry and attribute data such as rules of the road, vehicle height restrictions, lane count and centreline as well as curvature of intersections.
Jørgen Behrens regards Here Lanes as a “visualisation derivative” of the HD maps, which were primarily designed to help autonomous driving systems make decisions on braking, turning or moving to another lane on a highway.
“We have mapped the road network with higher density and so we know when you get to a complex junction, exactly how many lanes there are and how many arrows are drawn on the road,” he continued. “And so we are now making that available for people to use in the visualisation of the map in the navigation system, which is another purpose for the same data.
“We’ve essentially created a product that allows OEMs to visualise complex junctions, visualise the road with a higher level of realism so it looks like the real road on your navigation system,” Behrens concluded.
Here Last Mile
Here Technologies says Here Last Mile generates and distributes plans to maximise the efficient utilisation of an entire fleet of vehicles, considering multiple constraints such as delivery windows and cost.
The application is expected to help drivers find the best route to enable an on-time delivery by providing turn-by-turn navigation with real-time information on traffic flow and incidents and proof of delivery. Dynamic re-planning helps react to last-minute changes including a customer not being able to receive their parcel or the breakdown of a vehicle to propose the most efficient route at any given point in time, the company adds.
According to Here, the Tour Planning product has been equipped to Last Mile to optimise routes for the entire fleet taking into account delivery windows, job requirements, vehicle capabilities such as capacity and maximum range as well as traffic.
Here Last Mile can also be used offline, allowing the driver to keep navigating and updating the delivery status even in areas of low connectivity such as indoor environments or urban canyons.