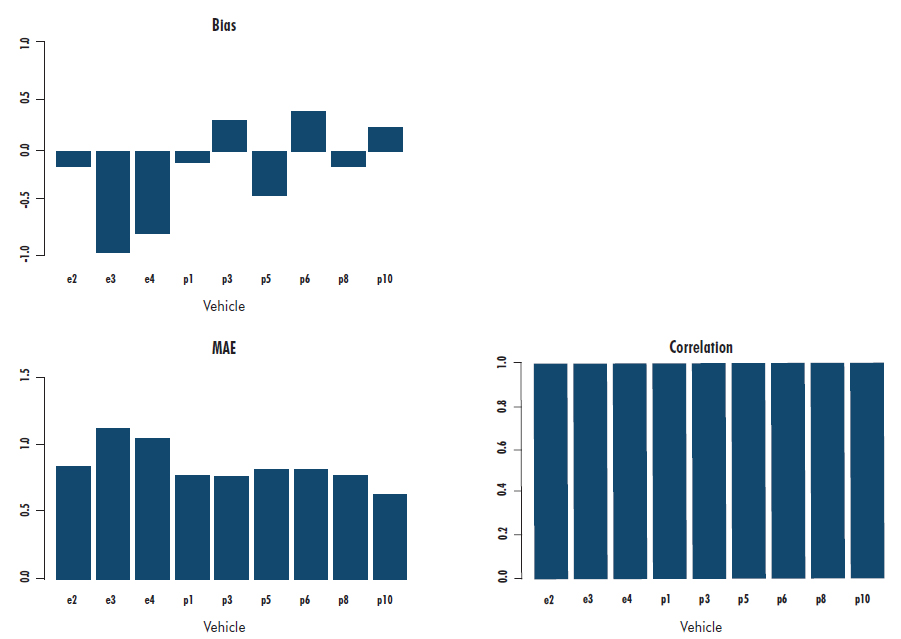
Sheldon Drobot, Michael Chapman and Amanda Anderson, NCAR, and Paul Pisano, FHWA, detail latest results of testing of a vehicle data translator for road weather monitoring and information applications
The use of vehicle sensor data to improve weather and road condition products, envisioned as part of theWith funding and support from RITA and direction from the
Test systems
The initial research was conducted at the Development Test Environment (DTE) facility located near Detroit, Michigan using six In addition to these sensors, temperature, dewpoint and surface temperature data were collected by
Ensuring accuracy
One of the initial questions the researchers faced centred on the accuracy and bias of in situ vehicle temperature and pressure measurements. After quality checking the observations, and using well-calibrated meteorological instruments as a validation source, comparisons indicate strong correspondence between quality check-passed data from the nine test vehicles and the in situ sensor for air temperature measurements, but some disparity for barometric pressure.Correlations are high in both cases (Table 1), but the bias and Mean Absolute Error (MAE) are far superior for temperature, indicating greater confidence in the temperature measurements. Without quality checking, the results degrade rapidly, particularly for pressure. In fact, for some vehicles more than half of the pressure observations failed the quality check procedure and if these data were used in the accuracy and bias computations, errors for some vehicles would exceed 30hPa with even larger bias numbers. Additional research is needed to ascertain precisely why the pressure data is subject to large errors but it clearly points to the need for rigorous quality checking of the raw data.
The researchers were also interested in determining whether variations in ambient conditions (for example, ambient air temperature variations, and precipitation versus no precipitation) or vehicle factors (such as vehicle speed or model) altered the overall results. If so, this would make the interpretation and use of mobile data a more complex operation.
Fortunately, there is little evidence that environmental factors alter the statistics shown in Table 1 (again, once the data are quality-checked). When stratified by ambient air temperature, time of day, day, wind direction and speed, or precipitation condition, there are more appreciable differences across the stratifications. Unfortunately, there is some evidence to suggest vehicles do introduce unique factors, though not for every condition. For example, error rates were consistent no matter what the vehicle speed, but when stratifying the statistics by vehicle (Figure 1 and Figure 2), some differences between vehicles are seen, particularly regarding bias.
The temperature bias shows variation not only between make of vehicle (Ford Edge versus Jeep Grand Cherokee), but also variation between vehicles of the same make. The MAE shows slightly less variation, with the Fords having a slightly higher MAE than the Jeeps. Correlation is high among all vehicles. For pressure, all vehicles exhibit a negative bias. Other non-environmental factors, such as vehicle colour and vehicle speed, had little demonstrable impact on the results.
Representativeness
One of the outstanding remaining questions revolves around the representativeness of these results for other makes and models. Additional research will be needed to determine that. Nonetheless, with confidence that these data are reliable, the researchers have spent time developing a suite of algorithms to provide drivers with road hazard warnings.These include 'road precipitation', which uses a combination of ambient air temperature, radar reflectivity, windshield wiper status, headlight status and the ratio of vehicle speed to road segment speed limit to determine what type of precipitation is influencing the roadway. Other algorithms include 'pavement slickness potential', 'visibility', and an 'all hazards' algorithm combining output from the three individual algorithms.
Currently, the output is displayed on a website with a
Now that the VDT has been successfully tested, NCAR and the USDOT are moving toward increasing the capabilities of the VDT. If you would like more information on VDT development, please contact Sheldon Drobot
(+1 303 497 2705;
Another feature of the VDT system is the incorporation of
Temperature (°C) | Pressure (hPa) | |
Bias | -0.21 | -4.33 |
MAE | 0.84 | 4.95 |
Correlation | 0.99 | 0.91 |
References
• Drobot, S.D., W.P. Mahoney III, P.A. Pisano, and B.B. McKeever, 2009: Tomorrow's Forecast: Informed Drivers.
• ITS International, July-August 2009, pp.NA1-NA2.
• Mitretek, 2006: Vehicles as Mobile Sensing Platforms for Meteorological Observations: Introductory Research during a Winter Season. 267 pp.